This website uses cookies so that we can provide you with the best user experience possible. Cookie information is stored in your browser and performs functions such as recognising you when you return to our website and helping our team to understand which sections of the website you find most interesting and useful.
Understanding Natural Language Processing: Revolutionizing Human-Machine Interaction
April 30, 2024 | by aiworldblog.com
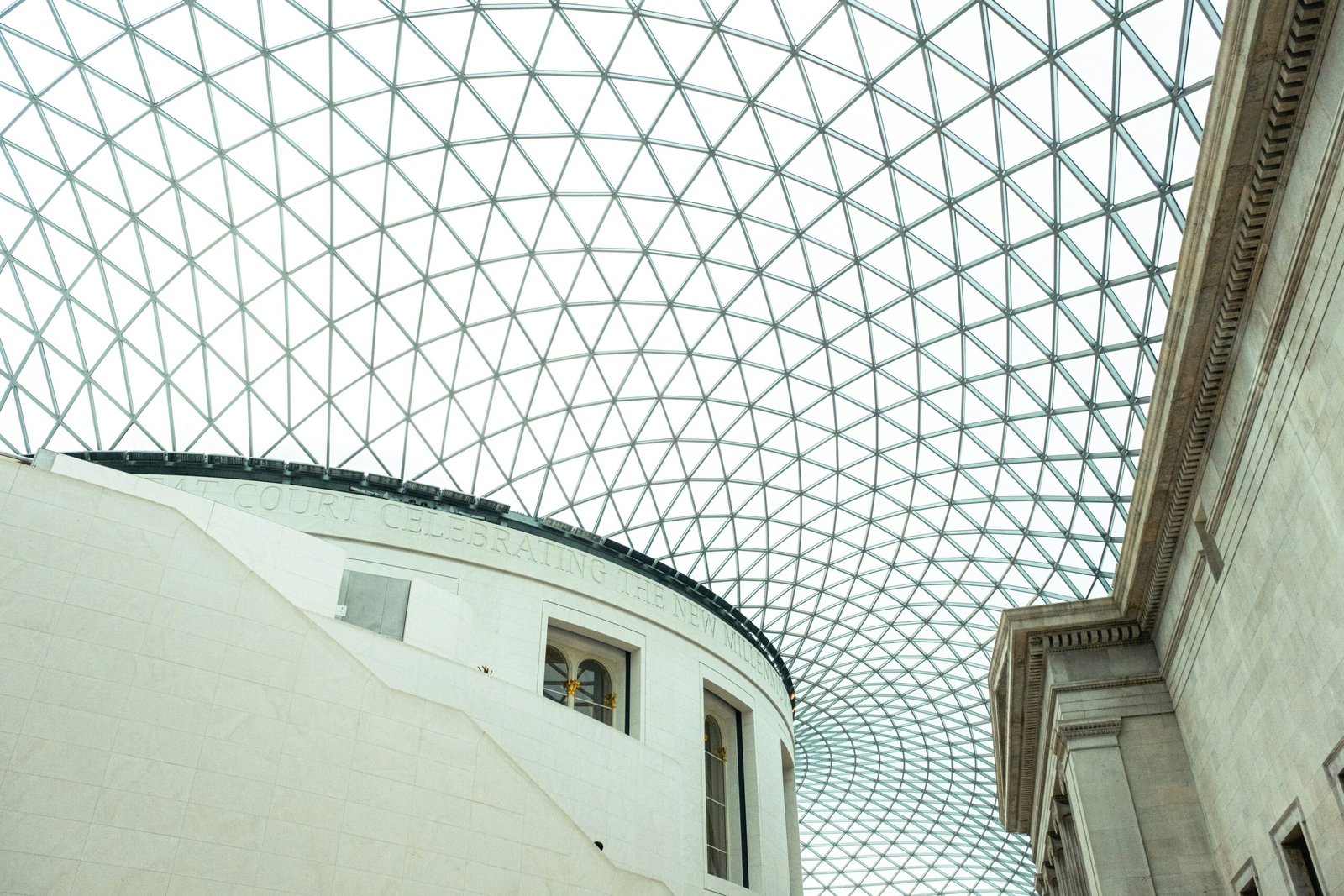
Natural Language Processing (NLP) is a subfield of Artificial Intelligence (AI) that focuses on the interaction between computers and human language. It involves the development of algorithms and models that enable machines to understand, interpret, and generate human language in a way that is similar to how humans do.
At its core, NLP aims to bridge the gap between human language and computer language. It involves the use of computational linguistics, machine learning, and other AI techniques to process and analyze vast amounts of text data. By doing so, NLP enables machines to extract meaning, sentiment, and context from text, allowing them to understand and respond to human language in a more intelligent and natural way.
One of the key challenges in NLP is the ambiguity and complexity of human language. Words and phrases can have multiple meanings depending on the context in which they are used, and understanding the intended meaning requires a deep understanding of language and its nuances. For example, the word “bank” can refer to a financial institution or the edge of a river, and determining the correct meaning requires considering the context in which it is used.
To tackle this challenge, NLP relies on various techniques such as syntactic and semantic analysis, named entity recognition, and sentiment analysis. These techniques allow machines to break down sentences into their constituent parts, identify the relationships between words, and extract relevant information. By analyzing the structure and meaning of sentences, NLP algorithms can derive insights and make intelligent decisions based on the input text.
NLP has a wide range of applications across different industries. In customer service, NLP-powered chatbots can understand and respond to customer queries, providing instant support and reducing the need for human intervention. In healthcare, NLP can be used to analyze medical records and extract valuable insights, improving diagnosis and treatment. In finance, NLP algorithms can analyze news articles and social media data to predict market trends and make informed investment decisions.
However, despite its advancements, NLP still faces several challenges. Understanding the nuances and subtleties of human language is a complex task, and NLP algorithms often struggle with sarcasm, irony, and other forms of figurative speech. Additionally, NLP models require large amounts of training data, which can be difficult to obtain for certain languages or domains.
Nevertheless, NLP continues to evolve and improve, driven by advancements in AI and machine learning. As technology progresses, we can expect NLP to play an even more significant role in our daily lives, enabling machines to understand and communicate with us in a more natural and human-like manner.
Another important task in Natural Language Processing is Named Entity Recognition. Named Entity Recognition (NER) is the process of identifying and classifying named entities in text into predefined categories such as person names, organizations, locations, dates, and more. This task is crucial for various applications, such as information extraction, question answering systems, and sentiment analysis.
NER algorithms typically use machine learning techniques to train models on annotated data. These models learn to recognize patterns and features in the text that indicate the presence of named entities. They can then be used to automatically identify and classify named entities in new, unseen text.
For example, consider the sentence: “Apple Inc. is planning to open a new store in London next month.” A NER system would recognize “Apple Inc.” as an organization, “London” as a location, and “next month” as a date. This information can be valuable for various applications, such as tracking company expansions, analyzing market trends, or providing personalized recommendations based on user preferences.
In addition to named entity recognition, another important task in NLP is coreference resolution. Coreference resolution is the process of determining when two or more expressions in a text refer to the same entity. This task is crucial for understanding the relationships between different entities and maintaining coherence in discourse.
For example, consider the following sentences: “John went to the store. He bought some groceries.” In this case, coreference resolution would identify that “John” and “He” refer to the same person. This information can be used to improve machine understanding of text and enable more advanced applications, such as summarization, question answering, and dialogue systems.
Overall, Natural Language Processing encompasses a wide range of tasks that aim to enable machines to understand and interact with human language. These tasks, including named entity recognition and coreference resolution, are crucial for developing intelligent systems that can process and generate text in a way that is meaningful and contextually relevant.
Applications of NLP in Everyday Life
Natural Language Processing has become an integral part of our daily lives, impacting various industries and applications. Here are some examples of how NLP is used in everyday life:
Virtual Assistants
Virtual assistants like Siri, Alexa, and Google Assistant rely heavily on NLP to understand and respond to user commands. They can perform tasks such as setting reminders, answering questions, and even controlling smart home devices, all through natural language interactions.
Chatbots
Chatbots are becoming increasingly popular in customer service, providing quick and efficient responses to customer inquiries. NLP enables chatbots to understand and interpret customer queries, providing relevant information or directing them to the appropriate resources.
Language Translation
NLP has greatly improved the accuracy and fluency of machine translation systems. Platforms like Google Translate utilize advanced NLP algorithms to translate text from one language to another, making it easier for people to communicate and understand each other across different languages.
Social Media Analysis
NLP techniques are used to analyze social media data, allowing companies to gain insights into customer opinions, sentiments, and trends. By analyzing large volumes of social media posts, businesses can make data-driven decisions and improve their products or services based on customer feedback.
Content Generation
NLP models can generate human-like text, which has applications in various areas such as content creation, summarization, and storytelling. These models can generate coherent and contextually relevant text, reducing the time and effort required for certain writing tasks.
Additionally, NLP is also used in spam detection, where it helps filter out unwanted emails or messages by analyzing their content and identifying patterns commonly associated with spam. NLP algorithms can also be applied in sentiment analysis, where they can determine the sentiment expressed in a piece of text, whether it is positive, negative, or neutral. This can be useful in monitoring public opinion towards a brand or product.
Furthermore, NLP plays a crucial role in information extraction and knowledge discovery. By analyzing large amounts of text data, NLP algorithms can identify relevant information and extract key facts or entities. This can be particularly useful in fields such as healthcare, where NLP can assist in extracting medical information from patient records or research papers.
In the field of education, NLP can be used to develop intelligent tutoring systems that can provide personalized feedback and assistance to students. By analyzing students’ responses and understanding their language, NLP algorithms can identify areas of weakness and provide targeted recommendations for improvement.
Overall, the applications of NLP in everyday life are vast and continue to expand as technology advances. From virtual assistants to content generation, NLP is revolutionizing the way we interact with technology and improving various aspects of our daily lives.
The Future of NLP
Natural Language Processing is an ever-evolving field, with continuous advancements being made in AI and machine learning. The future of NLP holds exciting possibilities, including:
Improved Language Understanding
As AI models become more sophisticated and are trained on larger datasets, their language understanding capabilities will continue to improve. This means machines will be able to understand and respond to human language with even greater accuracy and nuance.
Imagine a future where virtual assistants can understand not just the words we say, but also the underlying meaning and intent behind them. This would enable more natural and meaningful interactions, where machines can provide personalized and contextually relevant responses. Whether it’s a chatbot helping you with customer support or a voice assistant understanding your commands, improved language understanding would revolutionize the way we interact with technology.
Enhanced Multilingual Capabilities
NLP systems will continue to improve their ability to handle multiple languages, making language translation and cross-lingual communication even more seamless. This will facilitate global collaboration and communication, breaking down language barriers.
Imagine a future where language is no longer a barrier to communication. With enhanced multilingual capabilities, NLP systems could effortlessly translate conversations in real-time, allowing people from different parts of the world to communicate seamlessly. This would not only facilitate business collaborations but also foster cultural exchange and understanding.
Deeper Contextual Understanding
Future NLP models will be able to understand language in a more contextual and nuanced manner. They will be able to recognize sarcasm, irony, and other forms of figurative language, enabling more accurate interpretation and response.
Imagine a future where machines can understand the subtle nuances of human language. They would be able to detect sarcasm in a tweet, understand the underlying sentiment in a customer review, or grasp the subtle humor in a conversation. This deeper contextual understanding would enable more accurate and meaningful interactions, leading to improved customer service, more effective content analysis, and enhanced sentiment analysis.
Ethical Considerations
As NLP becomes more powerful, ethical considerations will become increasingly important. It will be crucial to ensure that NLP systems are unbiased, respectful of privacy, and used in a responsible and transparent manner.
Imagine a future where NLP systems are designed with fairness and inclusivity in mind. They would be trained on diverse datasets, free from biases and stereotypes, to ensure that their responses are not influenced by discriminatory factors. Additionally, privacy concerns would be addressed, with transparent data handling practices and user consent being paramount. Ethical considerations would guide the development and deployment of NLP systems, ensuring that they are used to benefit society as a whole.
In conclusion, the future of NLP is incredibly promising. With improved language understanding, enhanced multilingual capabilities, deeper contextual understanding, and ethical considerations, NLP systems will revolutionize the way we communicate, collaborate, and interact with technology.
RELATED POSTS
View all