This website uses cookies so that we can provide you with the best user experience possible. Cookie information is stored in your browser and performs functions such as recognising you when you return to our website and helping our team to understand which sections of the website you find most interesting and useful.
The Importance of Explainable AI and its Future Directions
April 30, 2024 | by aiworldblog.com
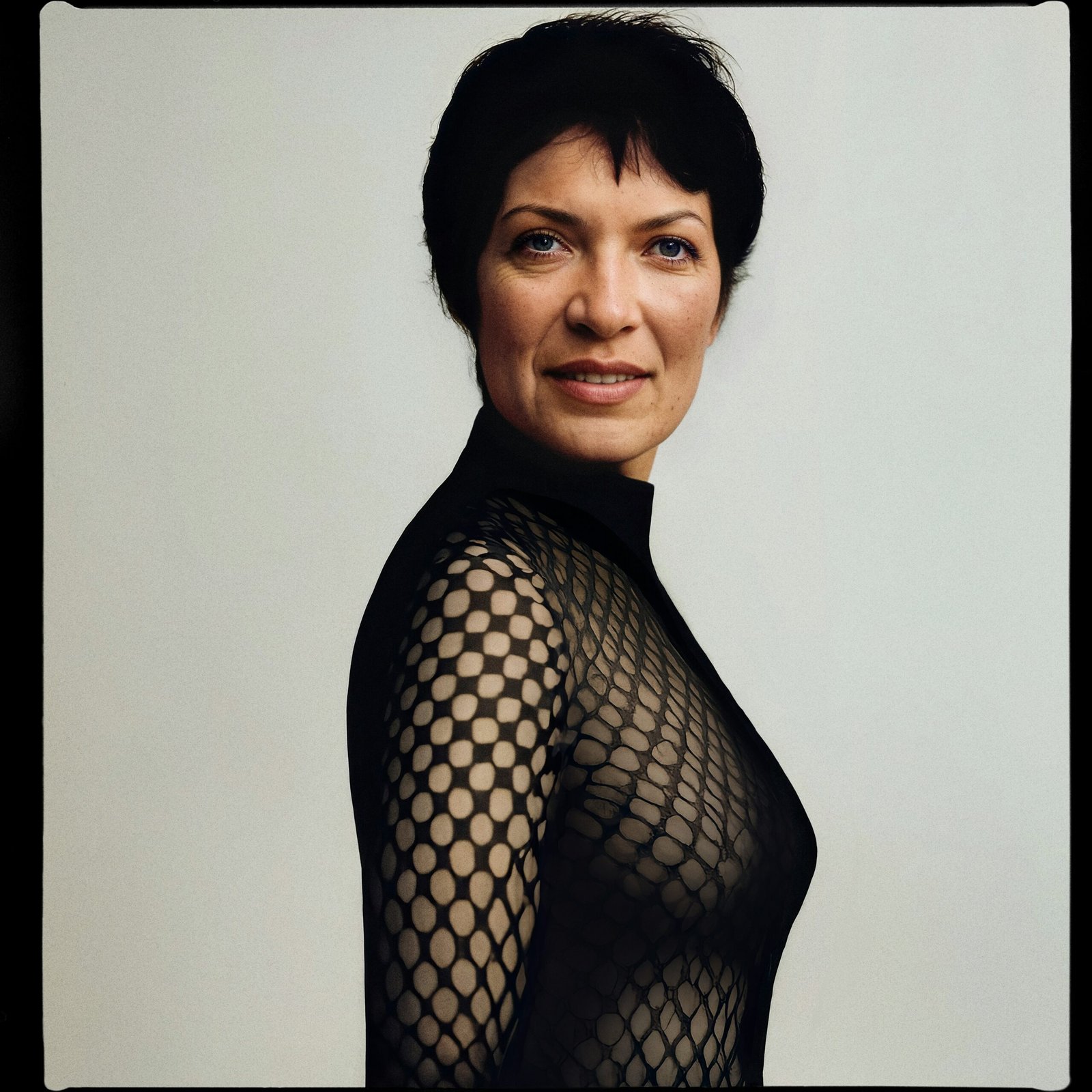
Artificial Intelligence (AI) is a branch of computer science that focuses on creating intelligent machines capable of performing tasks that typically require human intelligence. These tasks include speech recognition, decision-making, problem-solving, learning, and more. AI systems are designed to analyze large amounts of data, identify patterns, and make predictions or decisions based on that analysis.
Explaining Explainable AI
Explainable AI (XAI) is a subfield of AI that aims to make AI systems more transparent and understandable to humans. Traditional AI models, such as deep learning neural networks, often work as black boxes, making it difficult to understand how they arrive at their decisions. This lack of transparency can be problematic, especially in critical areas such as healthcare or finance, where the reasoning behind AI decisions is crucial.
Explainable AI focuses on developing AI systems that can provide clear explanations for their decisions or predictions. It aims to bridge the gap between the complex inner workings of AI models and human comprehension. By providing understandable explanations, XAI allows users to trust and validate AI systems, leading to increased acceptance and adoption.
One of the key challenges in XAI is striking a balance between transparency and performance. AI models that are highly transparent and explainable may sacrifice some degree of performance. On the other hand, models that prioritize performance may lack the ability to provide detailed explanations. Finding the right trade-off is crucial in developing effective explainable AI systems.
There are various techniques used in XAI to enhance the explainability of AI systems. One such technique is rule-based explanations, where the AI system generates a set of rules that explain its decision-making process. These rules can be easily understood by humans and provide insights into how the AI system arrived at a particular decision.
Another technique is feature importance analysis, which identifies the key features or variables that influenced the AI system’s decision. By highlighting the most influential factors, users can gain a better understanding of why the AI system made a specific prediction or decision.
Additionally, model-agnostic approaches in XAI aim to provide explanations that are applicable to different types of AI models. These approaches focus on extracting explanations from AI models without requiring access to their internal structure or parameters. By being model-agnostic, XAI techniques can be more widely applied and integrated into existing AI systems.
Explainable AI is not only important for building trust and understanding between humans and AI systems, but it also plays a crucial role in ensuring ethical and responsible AI deployment. By providing explanations, AI systems can be held accountable for their decisions and potential biases can be identified and addressed.
In conclusion, explainable AI is a field that seeks to make AI systems more transparent and understandable. Through techniques such as rule-based explanations, feature importance analysis, and model-agnostic approaches, XAI aims to bridge the gap between the inner workings of AI models and human comprehension. By providing clear explanations, XAI enhances trust, validation, and acceptance of AI systems, while also promoting ethical and responsible AI deployment.
5. Enhancing User Experience
Explainable AI plays a crucial role in enhancing the user experience. When users interact with AI systems, they often want to understand why certain recommendations or decisions are being made. By providing explanations, AI systems can empower users to make informed choices and have a deeper understanding of the system’s capabilities and limitations. This not only improves user satisfaction but also increases the adoption and acceptance of AI technology.
6. Facilitating Error Detection and Improvement
Explainable AI facilitates error detection and improvement by allowing developers and users to identify potential flaws or biases in the system’s decision-making process. When AI systems provide explanations, it becomes easier to pinpoint errors or inconsistencies in the data or algorithms. This enables developers to refine and improve the AI models, making them more accurate, reliable, and robust.
7. Ethical Considerations
Explainable AI is crucial from an ethical standpoint. As AI systems become more prevalent in various domains, it is essential to ensure that their decision-making processes align with ethical principles and values. By providing explanations, AI systems can be evaluated for fairness, transparency, and accountability. This helps in addressing ethical concerns such as privacy, bias, and discrimination, and promotes the development and deployment of AI technology that aligns with societal values.
8. Education and Understanding
Explainable AI promotes education and understanding of AI technology. By providing explanations, AI systems can help users, policymakers, and the general public gain insights into the underlying mechanisms and processes of AI. This fosters a greater understanding of AI technology and its potential impact on society. It also enables individuals to make informed decisions about the use and adoption of AI systems in various domains.
6. Model-agnostic methods
Model-agnostic methods in Explainable AI aim to provide transparency and interpretability across different types of AI models. These methods do not rely on the internal workings of a specific model and can be applied to any black box model. By analyzing the input-output relationship of the model, model-agnostic methods generate explanations that help humans understand how the model arrives at its decisions.
7. Counterfactual explanations
Counterfactual explanations provide insights into what changes in the input features would lead to a different outcome. By generating alternative scenarios and showing how the model’s decision would change, counterfactual explanations help humans understand the sensitivity of the model to different inputs. This allows for a better understanding of the decision-making process and can help identify potential biases or shortcomings in the model.
8. Certainty and confidence estimation
Estimating the certainty and confidence of AI models is crucial for understanding their reliability and potential limitations. Techniques such as uncertainty quantification and confidence estimation provide measures of the model’s confidence in its predictions. This information can be used to assess the reliability of the model’s decisions and identify cases where human intervention or further investigation may be necessary.
9. Interactive visualizations
Interactive visualizations allow users to explore and interact with the AI model’s decision-making process. These visualizations can provide insights into the model’s internal workings, highlight important features, and allow users to test different scenarios and observe the model’s response. By enabling users to actively engage with the model, interactive visualizations enhance understanding and trust in the AI system.
10. Ethical considerations
Explainable AI also takes into account ethical considerations to ensure that the explanations provided are fair, unbiased, and respectful of privacy. Ethical guidelines and frameworks are developed to address issues such as transparency, accountability, and the potential impact of AI systems on individuals and society. By incorporating ethical considerations into the design and implementation of AI systems, Explainable AI aims to promote responsible and trustworthy AI applications.
Challenges and Future Directions
While Explainable AI has made significant progress, there are still challenges to overcome and avenues for future research:
1. Balancing Transparency and Performance
There is often a trade-off between the transparency of AI systems and their performance. Highly transparent models, such as rule-based systems, may sacrifice performance compared to more complex black box models. Finding the right balance between transparency and performance is a challenge that researchers and developers continue to work on.
One approach to addressing this challenge is through the development of hybrid models that combine the interpretability of rule-based systems with the performance of black box models. By incorporating explainable components into complex models, researchers aim to achieve a balance between transparency and performance.
2. Scalability to Complex Models
Many current explainable AI techniques are designed for simpler models and may struggle to provide meaningful explanations for complex deep learning models. Developing techniques that can scale to complex models without sacrificing interpretability is an ongoing area of research.
One direction for future research is to explore the use of model-agnostic techniques that can provide explanations for a wide range of models, regardless of their complexity. By developing methods that are not tied to specific model architectures, researchers can ensure that explainability is achievable even for the most complex AI systems.
3. User Understanding and Interaction
Ensuring that users can understand and effectively interact with AI explanations is crucial. Designing user-friendly interfaces and visualization techniques that facilitate comprehension and decision-making is an important direction for future research.
Researchers are exploring various approaches to improve user understanding and interaction with AI explanations. This includes developing interactive visualizations that allow users to explore different aspects of the model’s decision-making process, as well as providing natural language explanations that are easier for non-technical users to comprehend.
4. Ethical Considerations
As AI becomes increasingly integrated into various aspects of society, ethical considerations become paramount. Ensuring that AI systems are fair, unbiased, and respect privacy is a challenge that must be addressed in the development and deployment of explainable AI systems.
Researchers and policymakers are actively working on developing guidelines and frameworks to address these ethical considerations. This includes promoting transparency in AI systems, implementing mechanisms for auditing and accountability, and ensuring that AI systems are designed and trained on diverse and representative datasets to mitigate biases.
In conclusion, while Explainable AI has made significant strides, there are still challenges to overcome and areas for future research. By addressing these challenges and considering the ethical implications, we can continue to advance the field of explainable AI and ensure that AI systems are not only capable of making accurate predictions but also provide understandable and trustworthy explanations for their decisions.
RELATED POSTS
View all