This website uses cookies so that we can provide you with the best user experience possible. Cookie information is stored in your browser and performs functions such as recognising you when you return to our website and helping our team to understand which sections of the website you find most interesting and useful.
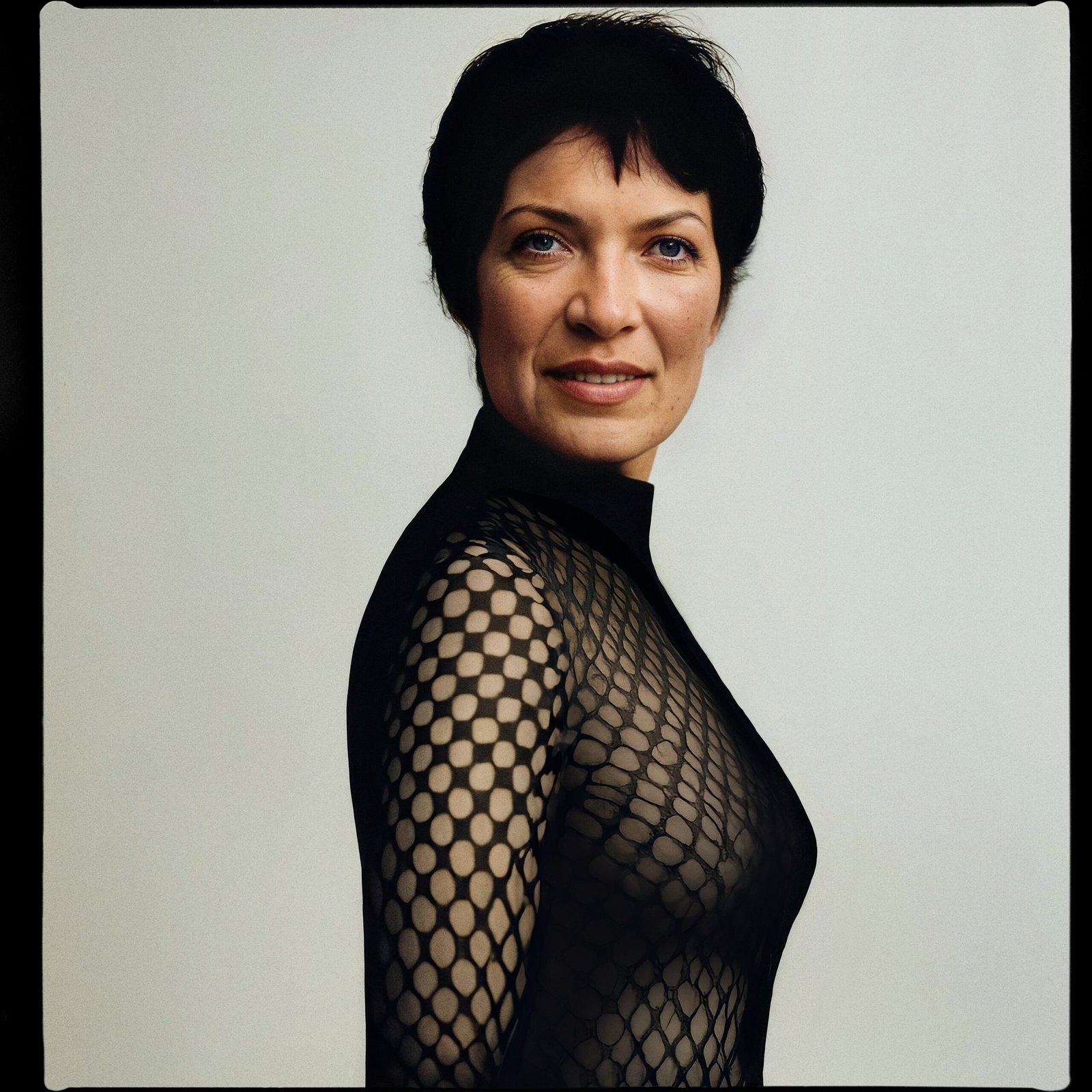
What is Artificial Intelligence Deep Learning?
Artificial Intelligence (AI) is a branch of computer science that focuses on creating intelligent machines capable of performing tasks that typically require human intelligence. Deep learning, on the other hand, is a subset of AI that is inspired by the structure and function of the human brain.
Deep learning algorithms are designed to learn and make predictions or decisions by analyzing large amounts of data. These algorithms are composed of artificial neural networks that are capable of automatically learning and improving from experience without being explicitly programmed.
Deep learning has gained significant attention and popularity in recent years due to its ability to solve complex problems in various domains. One of the key advantages of deep learning is its ability to extract meaningful features from raw data, which allows it to automatically learn and adapt to new information.
Deep learning algorithms rely on neural networks, which are composed of interconnected nodes or “neurons”. These neurons are organized into layers, with each layer responsible for extracting different levels of abstraction from the input data. The input data is fed into the network, and as it passes through the layers, the network progressively learns to recognize patterns and make predictions.
One of the reasons why deep learning has gained so much attention is its success in image and speech recognition tasks. Deep learning algorithms have achieved state-of-the-art performance in tasks such as object detection, facial recognition, and natural language processing. For example, deep learning models have been able to surpass human performance in tasks like image classification and speech recognition.
Deep learning has also found applications in fields such as healthcare, finance, and autonomous vehicles. In healthcare, deep learning models have been used to analyze medical images and detect diseases such as cancer. In finance, deep learning algorithms have been employed to predict stock prices and make investment decisions. In autonomous vehicles, deep learning is used to analyze sensor data and make real-time decisions for safe navigation.
However, deep learning is not without its challenges. One of the main challenges is the need for large amounts of labeled data for training. Deep learning models require vast amounts of data to learn effectively, and acquiring and labeling this data can be time-consuming and expensive. Additionally, deep learning models can be computationally intensive and require powerful hardware to train and deploy.
Despite these challenges, deep learning continues to advance rapidly, and its potential for solving complex problems is only just beginning to be realized. As researchers and engineers continue to push the boundaries of deep learning, we can expect to see even more impressive applications and breakthroughs in the future.
The Basics of Deep Learning
Deep learning is based on the concept of artificial neural networks, which are designed to mimic the structure and function of the human brain. These networks consist of interconnected layers of artificial neurons, also known as nodes or units.
Each node in a neural network receives input signals, processes them using an activation function, and produces an output signal. The output signal is then passed on to the next layer of nodes, forming a hierarchical structure.
The key feature of deep learning is the ability to automatically learn and extract meaningful features from raw data. This is achieved through a process called training, where the neural network is exposed to a large dataset and adjusts its internal parameters to minimize the difference between its predictions and the true values.
During the training process, the neural network learns to recognize patterns and relationships in the data by adjusting the weights and biases of its nodes. These weights and biases determine the strength of the connections between nodes and the activation threshold of each node, respectively.
Once the neural network has been trained, it can be used to make predictions on new, unseen data. This is known as inference. The network takes the input data, passes it through its layers of nodes, and produces an output that represents its prediction or classification.
Deep learning has revolutionized many fields, including computer vision, natural language processing, and speech recognition. It has achieved state-of-the-art performance in tasks such as image classification, object detection, and machine translation.
One of the reasons deep learning has been so successful is its ability to handle large amounts of data. The more data the network is exposed to during training, the better it becomes at generalizing and making accurate predictions on unseen data.
However, deep learning also has its limitations. It requires a significant amount of computational power and memory to train and run large neural networks. Additionally, the lack of interpretability of deep learning models makes it difficult to understand the reasoning behind their predictions.
Despite these challenges, deep learning continues to advance and push the boundaries of what is possible in artificial intelligence. Researchers are constantly developing new architectures, algorithms, and techniques to improve the performance and efficiency of deep learning models.
Medical Diagnosis
Deep learning has shown great potential in the field of medical diagnosis. By analyzing large amounts of medical data, deep learning algorithms can assist doctors in making accurate diagnoses and predicting patient outcomes. For example, deep learning models have been developed to detect early signs of diseases such as cancer, Alzheimer’s, and heart disease, allowing for earlier intervention and treatment.
Autonomous Vehicles
Deep learning has played a crucial role in the development of autonomous vehicles. With the help of deep neural networks, self-driving cars can perceive and interpret their surroundings, making decisions in real-time. Deep learning algorithms can process data from various sensors, such as cameras and LiDAR, to detect objects, predict their movements, and navigate safely on the road. This technology has the potential to revolutionize transportation and make our roads safer.
Recommendation Systems
Deep learning has greatly improved recommendation systems, which are used in various industries to personalize user experiences and provide relevant suggestions. By analyzing user behavior and preferences, deep learning models can accurately recommend products, movies, music, and more. These algorithms can understand complex patterns and make predictions based on large amounts of data, leading to better user satisfaction and increased sales for businesses.
Financial Forecasting
Deep learning has been applied to financial forecasting, helping investors and financial institutions make more informed decisions. By analyzing historical market data, deep learning models can predict stock prices, identify market trends, and detect anomalies. These models can process vast amounts of data and consider various factors, such as news sentiment, economic indicators, and market volatility, to provide valuable insights for investment strategies.
Robotics
Deep learning has also made significant contributions to robotics. By combining deep neural networks with robotics systems, robots can perceive their environment, understand human gestures and speech, and perform complex tasks. Deep learning algorithms enable robots to learn from experience and adapt to different situations, making them more intelligent and versatile. This has implications for industries such as manufacturing, healthcare, and logistics.
Security and Fraud Detection
Deep learning has been instrumental in enhancing security systems and fraud detection methods. Deep neural networks can analyze patterns and anomalies in large datasets to identify potential threats and fraudulent activities. These algorithms can be used for tasks such as facial recognition, biometric authentication, and credit card fraud detection. By leveraging deep learning, organizations can strengthen their security measures and protect sensitive information.
Environmental Monitoring
Deep learning has also found applications in environmental monitoring and conservation efforts. By analyzing satellite imagery and other environmental data, deep learning models can identify and track changes in ecosystems, detect deforestation, monitor wildlife populations, and predict natural disasters. These insights can help researchers, conservationists, and policymakers make informed decisions to protect and preserve our environment.
Continual Learning and Lifelong AI
Continual learning and lifelong AI are emerging areas of research that aim to enable deep learning models to learn and adapt over time. Traditional deep learning models are typically trained on fixed datasets and require retraining whenever new data is introduced. However, in real-world scenarios, data is constantly changing and evolving. Continual learning and lifelong AI seek to develop models that can learn from new data while retaining previously learned knowledge, allowing them to continuously improve and adapt to new situations.
Robustness and Adversarial Attacks
Robustness is a critical aspect of deep learning models, as they need to perform well even in the presence of noisy or adversarial inputs. Adversarial attacks involve intentionally manipulating input data to deceive deep learning models and cause them to make incorrect predictions. Researchers are working on developing robust deep learning models that are resistant to such attacks, ensuring the reliability and security of AI systems in real-world applications.
Human-AI Collaboration
The future of AI deep learning also involves exploring ways to facilitate effective collaboration between humans and AI systems. This includes developing interfaces and tools that allow humans to interact with and understand AI models, as well as designing AI systems that can effectively communicate and collaborate with humans. Human-AI collaboration has the potential to enhance decision-making, creativity, and problem-solving capabilities, leading to more impactful and beneficial applications of AI in various domains.
Hardware and Efficiency
As deep learning models become larger and more complex, there is a need for efficient hardware solutions to support their training and inference. Researchers are exploring new hardware architectures, such as specialized AI chips and neuromorphic computing, to improve the speed and energy efficiency of deep learning computations. Advancements in hardware technology will play a crucial role in enabling the deployment of AI systems in resource-constrained environments and accelerating the progress of AI research.
Interdisciplinary Approaches
The future of AI deep learning lies in embracing interdisciplinary approaches that combine insights from various fields. Collaboration between computer science, neuroscience, psychology, and other disciplines can lead to breakthroughs in understanding the human brain, developing more biologically inspired AI models, and advancing our understanding of intelligence. By bridging the gap between different disciplines, researchers can unlock new possibilities and drive innovation in the field of AI deep learning.
RELATED POSTS
View all